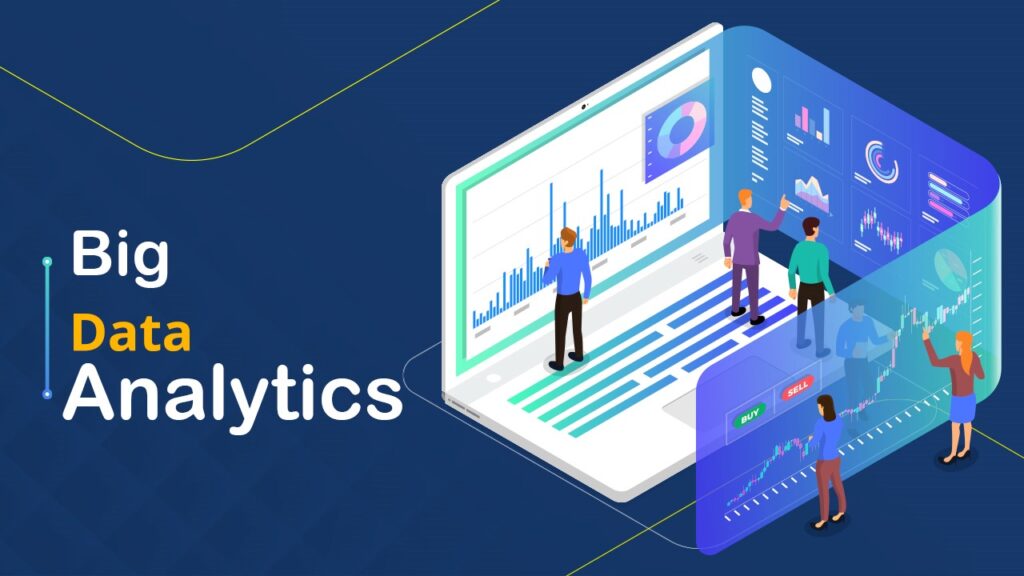
Introduction
In today’s data-driven world, making informed business decisions is crucial for success. This is where big data analytics plays a significant role. Big data analytics involves extracting valuable insights from vast and complex datasets to drive strategic decision making. By analyzing large volumes of data from diverse sources, businesses can gain a deeper understanding of customer behavior, market trends, and operational efficiencies. This enables companies to make data-driven decisions that lead to improved outcomes, such as enhanced customer experiences, optimized processes, and increased profitability. In this blog post, we will explore the transformative power of big data analytics and how it empowers businesses to make smarter, more informed decisions.
Overview of Big Data Analytics
Big data analytics is a process of extracting actionable insights from large and complex datasets. With the ever-increasing volume, velocity, and variety of data being generated, organizations are leveraging big data analytics to gain valuable insights and make informed business decisions.
The process of big data analytics involves several stages. First, data is collected from various sources, including structured and unstructured data, such as customer transactions, social media posts, sensor data, and more. This data is then stored in data repositories or data lakes for further processing.
Next, the collected data is processed and analyzed using advanced analytics techniques. This includes data cleaning, transformation, and aggregation to ensure the data is in a usable format. Analytical tools and algorithms are applied to uncover patterns, trends, and correlations within the data.
There are different types of big data analytics: descriptive, predictive, and prescriptive. Descriptive analytics focuses on summarizing historical data to provide insights into past events and trends. Predictive analytics utilizes statistical models and machine learning algorithms to forecast future outcomes based on historical data patterns. Prescriptive analytics goes a step further by recommending the best course of action based on predictive models and business objectives.
The benefits of big data analytics in business are significant. It enables organizations to gain a deeper understanding of their customers, allowing for personalized marketing campaigns, improved customer experiences, and targeted product development. It also helps identify market trends and opportunities, facilitating proactive decision-making. In addition, big data analytics enhances operational efficiency by optimizing processes, reducing costs, and mitigating risks. From supply chain optimization to fraud detection and predictive maintenance, big data analytics empowers organizations to make data-driven decisions that drive growth and competitiveness.
Overall, big data analytics provides organizations with the capability to turn vast amounts of data into valuable insights, empowering them to make informed decisions that can positively impact their business.
Benefits of Big Data Analytics in Business Decision Making
Improved decision-making through data-driven insights:
Big data analytics enables businesses to base their decisions on solid evidence and insights derived from analyzing large volumes of data. By analyzing historical and real-time data, businesses can identify patterns, trends, and correlations that may not be apparent through traditional decision-making processes. This data-driven approach reduces the reliance on intuition or guesswork and allows organizations to make more accurate and informed decisions. For example, analyzing customer behavior data can help businesses optimize their marketing strategies, product offerings, and pricing strategies to better meet customer needs and preferences.
Enhanced understanding of customer behavior and preferences:
One of the key benefits of big data analytics is the ability to gain deep insights into customer behavior and preferences. By analyzing customer data, such as purchase history, browsing patterns, and social media interactions, businesses can identify patterns and trends that can guide their marketing and sales strategies. This understanding enables businesses to personalize their offerings, target specific customer segments, and provide tailored experiences. For instance, an e-commerce company can use big data analytics to recommend relevant products to customers based on their browsing and purchase history, increasing the chances of conversions and customer satisfaction.
Identification of market trends and opportunities:
Big data analytics allows businesses to stay ahead of the competition by identifying emerging market trends and opportunities. By analyzing data from various sources, including social media, online reviews, and industry reports, organizations can spot market shifts, consumer preferences, and new demands. This knowledge enables businesses to adapt their strategies, develop innovative products, and enter new markets. For example, a retail company can use big data analytics to identify rising consumer trends and adjust its product offerings accordingly, gaining a competitive advantage and capturing new market segments.
Increased operational efficiency and cost savings:
Big data analytics can help optimize business operations and reduce costs. By analyzing operational data, organizations can identify inefficiencies, bottlenecks, and areas for improvement. This insight allows businesses to streamline processes, allocate resources effectively, and reduce waste. For instance, a logistics company can analyze transportation data to optimize routes, reduce fuel consumption, and improve delivery schedules, resulting in cost savings and improved customer satisfaction.
Mitigation of risks and fraud detection:
Big data analytics plays a crucial role in risk management and fraud detection. By analyzing vast amounts of data, organizations can detect anomalies, patterns, and potential risks. This enables proactive risk mitigation and fraud prevention. For example, financial institutions can use big data analytics to detect suspicious transactions and identify potential fraudulent activities, safeguarding their customers’ assets and maintaining trust.
In conclusion, big data analytics provides numerous benefits for business decision-making. It empowers organizations to make informed decisions based on data-driven insights, understand customer behavior, identify market trends and opportunities, enhances operational efficiency, and mitigate risks. By harnessing the power of big data analytics, businesses can gain a competitive edge and drive growth in today’s data-centric business environment.
Use Cases of Big Data Analytics in Business
Big data analytics has a wide range of use cases across various industries. Here are some examples of how businesses can leverage big data analytics to drive growth and make informed decisions:
Customer segmentation and personalized marketing:
Big data analytics enables businesses to segment their customer base more effectively and tailor marketing strategies accordingly. By analyzing customer data such as demographics, purchase history, and browsing behavior, organizations can identify distinct customer segments and create personalized marketing campaigns. This approach allows businesses to deliver targeted messages, recommendations, and offers, resulting in higher customer engagement, conversion rates, and customer satisfaction.
Demand forecasting and inventory management:
Big data analytics can help businesses optimize their supply chain by accurately forecasting demand and improving inventory management. By analyzing historical sales data, seasonality patterns, market trends, and external factors like weather or economic indicators, organizations can predict future demand and adjust their production and inventory levels accordingly. This minimizes stockouts, reduces excess inventory costs, and improves overall operational efficiency.
Sentiment analysis and social media monitoring:
Big data analytics enables businesses to monitor and analyze customer sentiment and feedback on social media platforms. By analyzing social media posts, reviews, and comments, organizations can gain insights into customer opinions, preferences, and brand perception. This information helps businesses understand the strengths and weaknesses of their products or services, identify areas for improvement, and proactively address customer concerns or issues.
Predictive maintenance in manufacturing:
Big data analytics can play a crucial role in predictive maintenance for manufacturing industries. By collecting and analyzing sensor data from machinery and equipment, organizations can detect patterns and anomalies that indicate potential equipment failures or maintenance needs. This proactive approach allows businesses to schedule maintenance activities in advance, minimize unplanned downtime, reduce maintenance costs, and optimize overall equipment efficiency.
Fraud detection and prevention in financial services:
Big data analytics is instrumental in detecting and preventing fraudulent activities in the financial services industry. By analyzing large volumes of transactional data, organizations can identify patterns, anomalies, and suspicious behaviors that may indicate fraudulent activities. This helps businesses prevent financial losses, protect customer assets, and maintain the integrity of their systems and operations.
These use cases demonstrate the versatility of big data analytics in driving business outcomes. By leveraging data-driven insights, organizations can make informed decisions, improve customer experiences, optimize operations, and gain a competitive edge in their respective industries.
Challenges and Considerations in Implementing Big Data Analytics
Implementing big data analytics in an organization comes with its own set of challenges and considerations. Here are some key challenges and considerations businesses need to address:
Data quality and data governance issues:
One of the primary challenges in big data analytics is ensuring the quality and reliability of the data being analyzed. Data may come from various sources and in different formats, making it crucial to establish robust data governance practices. This involves implementing data validation processes, data cleansing techniques, and ensuring data integrity throughout the analytics pipeline. Organizations need to invest in data quality tools, establish data governance frameworks, and define data standards to address these challenges effectively.
Privacy and security concerns:
Big data analytics involves processing and analyzing large volumes of sensitive data, including customer information, financial data, and proprietary business data. Protecting data privacy and ensuring security are critical considerations. Organizations must comply with relevant data protection regulations and implement stringent security measures to safeguard data. This includes data encryption, access controls, user authentication, and implementing security protocols throughout the analytics infrastructure.
Technical infrastructure and scalability requirements:
Implementing big data analytics requires a robust technical infrastructure capable of handling large volumes of data and performing complex analytics processes. Organizations need to invest in scalable storage systems, high-performance computing resources, and distributed processing frameworks like Hadoop or Spark. Scalability considerations include the ability to handle growing data volumes, accommodate changing analytics requirements, and ensure efficient data processing and analysis.
Skills and talent acquisition for data analytics:
Effective implementation of big data analytics requires skilled professionals who understand both the technical aspects and the business context. There is a shortage of data scientists and analysts with the expertise to extract insights from big data. Organizations need to invest in hiring, training, and retaining skilled data professionals or consider partnering with external experts to overcome this talent gap.
Addressing these challenges and considerations requires careful planning and strategic decision-making. Organizations should develop a comprehensive data strategy, establish data governance frameworks, invest in robust infrastructure, ensure data privacy and security, and prioritize acquiring and developing the necessary skills and talent. By proactively addressing these challenges, businesses can maximize the benefits of big data analytics while mitigating potential risks.
Future Trends in Big Data Analytics
The field of big data analytics is continuously evolving, driven by advancements in technology and changing business needs. Here are some future trends that are expected to shape the landscape of big data analytics:
Advances in machine learning and artificial intelligence (AI):
Machine learning and AI techniques are poised to play a significant role in the future of big data analytics. These technologies can automate and enhance data analysis processes, enabling businesses to extract insights from large datasets more efficiently. As algorithms become more sophisticated and capable of handling complex patterns, businesses can expect more accurate predictions, improved anomaly detection, and enhanced decision support systems.
Integration of big data analytics with the Internet of Things (IoT):
The proliferation of IoT devices is generating vast amounts of data that can be leveraged for analytics. Big data analytics will increasingly be integrated with IoT systems to process and analyze real-time data streams from sensors, connected devices, and machines. This integration will enable organizations to gain valuable insights for optimizing operations, predicting maintenance needs, and delivering personalized experiences.
Ethical considerations in data analytics and AI:
As the use of big data analytics and AI grows, there will be a greater emphasis on ethical considerations. Organizations will need to address issues such as data privacy, bias in algorithms, and transparent decision-making. Stricter regulations and frameworks for responsible data usage and AI ethics are expected to emerge to ensure the ethical deployment of big data analytics.
Adoption of edge computing for real-time analytics:
Edge computing, which involves processing data closer to its source rather than sending it to centralized servers, is gaining traction. This approach reduces latency and enables real-time analytics at the edge of the network. By leveraging edge computing, organizations can make faster decisions, respond to events in real time, and reduce the need for extensive data transfers.
Focus on explainable AI and interpretable models:
As AI models become more complex, there is a growing need for explainability and interpretability. Businesses will seek to understand how AI models make decisions and provide transparent explanations. This will drive the development of techniques and algorithms that can provide insights into the decision-making process of AI models, ensuring transparency, fairness, and accountability.
These future trends in big data analytics indicate an exciting and transformative journey ahead. Organizations that embrace these trends and adapt their strategies accordingly will be well-positioned to leverage the power of big data analytics to drive innovation, gain a competitive edge, and make more informed decisions.
Conclusion
In conclusion, big data analytics is revolutionizing the way businesses operate and make decisions. By harnessing the power of large and complex datasets, organizations can gain valuable insights, improve customer experiences, optimize operations, and identify new opportunities. The future of big data analytics is marked by advancements in machine learning, integration with IoT, ethical considerations, edge computing, and the focus on explainable AI. As businesses embrace these trends and overcome challenges, they can unlock the full potential of big data analytics, stay ahead in a data-driven world, and drive growth and success in their respective industries.